Motivation
Understanding animal behavior, i.e., how they move and interact among each other and with their environment, is a fundamental requirement for addressing the most important ecological problems today. Fast and accurate understanding of animal behavior depends on their accurate 3D body pose and shape estimated over time. This estimation is called '4D motion capture', or MoCap. State-of-the-art methods for animal MoCap either require sensors or markers on the animals (e.g., GPS collars and IMU tags), or rely on camera traps fixed in the animal's environment. Not only do these methods pose danger to the animals due to tranquilization and physical interference, but their scope is also difficult to extend to a larger number of animals in vast environments. In WildCap, we are developing autonomous methods for MoCap of endangered wild animals, which will address the aforementioned issues. Our methods will not require any physical interference with the animals. Our novel approach is to develop a team of intelligent, autonomous and vision-based aerial robots which will detect, track, follow and perform MoCap of wild animals.
Ziele und Aufgaben
WildCap's goal is to achieve continuous, accurate and on-board 4D MoCap of domestic and endangered wild animal species from multiple, unsynchronized and close-range aerial images acquired in the animal's natural habitat, without any sensors or markers on the animal, and without modifying the environment.
In pursuit of the above goal, the key objectives of this project are
1. Development of novel aerial platforms for animal MoCap.
2. Formation control methods for multiple aerial robots tracking animals
3. Animal 4D MoCap (pose and shape over time) from multiple unsynchronized images.
Methodologie
Aerial robots with longer autonomy time and payload are critical for continuous and long distance tracking of animals in the wild. To this end, we are developing novel systems, particularly lighter than air vehicles that could potentially address these issues. Furthermore, we are developing formation control strategies for such vehicles to maximize the visual coverage of animals and accuracy in their state estimates. Finally, we are leveraging learning-in-simulation methods to develop algorithms for 4D pose and shape estimation of animals. The methodology will be updated once initial results from the project are obtained.
Publications
[1] Liu, Y.T., Price, E., Black, M.J., Ahmad, A. (2022) Deep Residual Reinforcement Learning based Autonomous Blimp Control, Accepted to IEEE/RSJ International Conference on Intelligent Robots and Systems (IROS), 2022.
[2] Saini, N., Bonetto, E., Price, E., Ahmad, A., & Black, M. J. (2022). AirPose: Multi-View Fusion Network for Aerial 3D Human Pose and Shape Estimation. IEEE Robotics and Automation Letters, 7(2), 4805–4812.https://doi.org/10.1109/LRA.2022.3145494
[3] Price, E., Liu, Y.T., Black, M.J., Ahmad, A. (2022). Simulation and Control of Deformable Autonomous Airships in Turbulent Wind. In: Ang Jr, M.H., Asama, H., Lin, W., Foong, S. (eds) Intelligent Autonomous Systems 16.IAS 2021. Lecture Notes in Networks and Systems, vol 412. Springer, Cham. https://doi.org/10.1007/978-3-030-95892-3_46
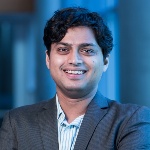
Aamir Ahmad
Jun.-Prof. Dr.-Ing.Deputy Director (Research)
Yu-Tang Liu
M. Sc.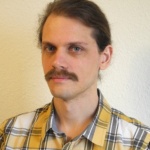